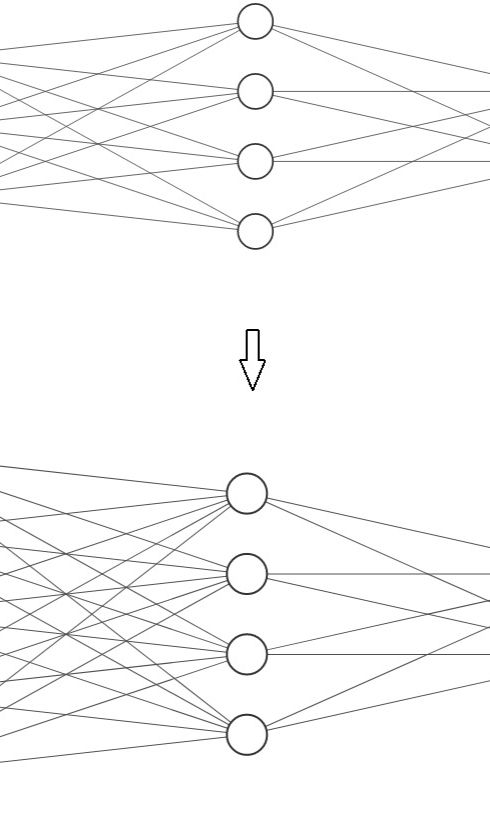
CANCER CELL DETECTION
Problem background
The client, a biotechnology company, required a machine vision solution to find potentially cancerous cells in microscopy slide scans as part of a clinical trial.
How the problem was approached
Once it was established what form the cell data took, an artificial neural network was designed, programmed and refined (see example iterations diagram, left) which was able to learn a baseline from a set of control data; data known to be from cancer-free cells (as validated by a human expert). The neural network was then shown many examples of defective cells of varying severity and was programmed to give a specific grading depending on the nature and magnitude of the issue detected.
Results
The network was able to effectively recognise, with greater-than-target accuracy, cells of concern and to what degree.
Key terms
-
Machine vision
-
Cell morphology analysis
-
malignancy-probability estimation.
-
Cytopathology
-
Machine vision
-
Bright-field microscopy
-
Specificity and sensitivity tuning
-
Biomedical research